【新技术】照片到视频,就像航拍一样柔滑:NeRF origina

北邦
发布于 2023-05-23 00:45
由原团队建造的NeRF在2023年取得了快速进展。
Built by the original team, https://aitool.ai/tag/nerf NeRF in 2023 has made rapid progress.
2020年,加州大学伯克利分校和谷歌的研究人员开启了一项将2D图像转换为3D模型NeRF的重要研究。它可以使用多个静态图像生成多视角的逼真3D图像,生成效果非常惊人:
In 2020, researchers from the University of California at Berkeley and Google opened an important research on converting 2D images into 3D models-NeRF. It can use several static images to generate realistic 3D images with multiple viewing angles, and the generation effect is very amazing:
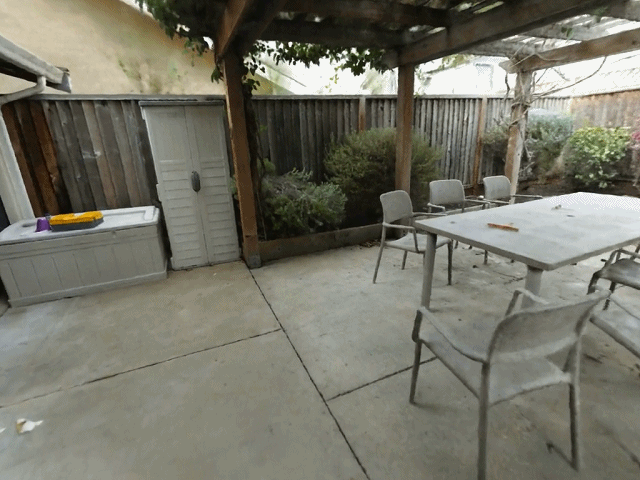
三年后,该团队做出了更惊人的效果:在一项名为Zip-NeRF的研究中,他们完全还原了一个家庭的所有场景,就像无人航拍的效果一样。
Three years later, the team made a more amazing effect: In a study called Zip-NeRF, they completely restored all the scenes of a family, just like the effect of unmanned aerial photography.
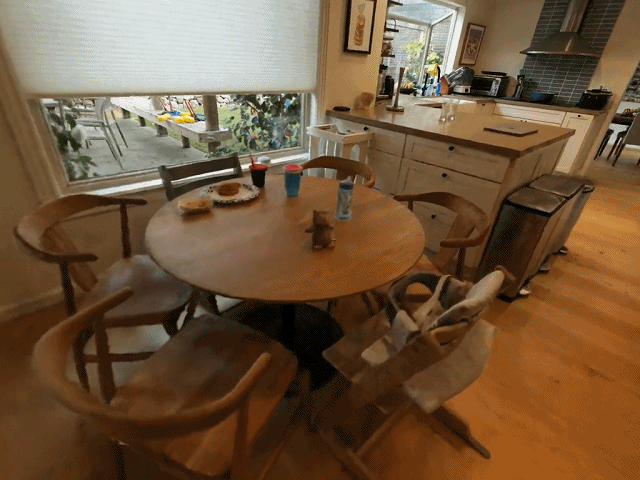
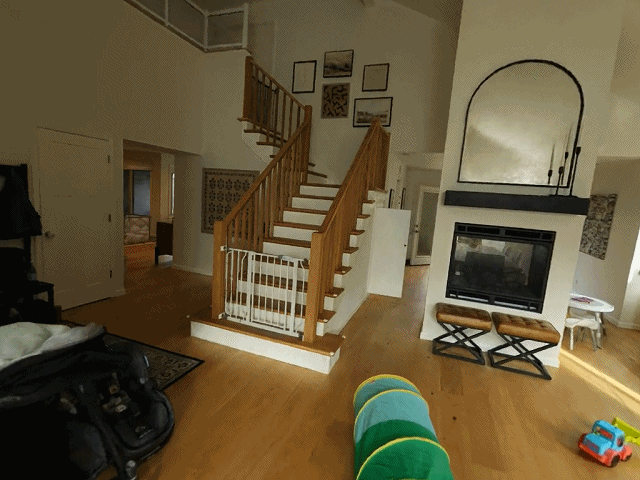
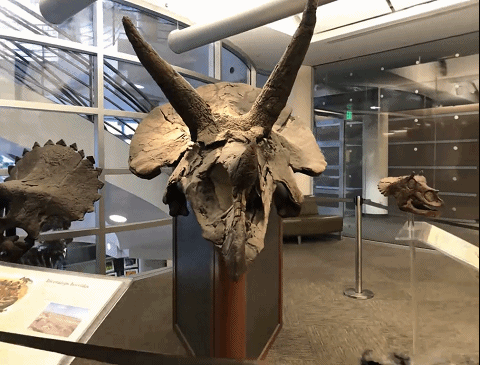
作者介绍了Zip-NeRF模型将尺度感知抗混叠NeRF和基于快速网格的NeRF训练相结合,解决了神经辐射场训练中的混叠问题。与以前的技术相比,Zip-NeRRF的错误率降低了8-76%,训练速度提高了22倍。
The author introduces that Zip-NeRF model combines scale-aware anti-aliasing NeRF and fast grid-based NeRF training to solve the aliasing problem in nerve radiation field training. Compared with previous technologies, the error rate of Zip-NeRF is reduced by 8-76%,The training speed is increased by 22 times.
这项技术有望应用于虚拟现实领域,例如参观在线博物馆和参观在线房屋。
This technology is expected to be applied in VR field, such as visiting online museums and visiting online houses.

以下是论文的详细内容。
The following are the details of the paper.

Paper address:
https://arxiv.org/pdf/2304.06706.pdf
Project address:
https://jonbarron.info/zipnerf/
论文概述 Paper Overview
在神经辐射领域(NeRF),训练神经网络来模拟三维场景的体积表示,以便通过光线跟踪呈现场景的新视图。NeRF已被证明是一种有效的任务工具,如视图合成、媒体生成、机器人和计算摄影。
In the neural radiation field (NeRF), a neural network is trained to simulate the volume representation of a three-dimensional scene, so that a new view of the scene can be presented through ray tracing. NeRF has proven to be an effective task tool, such as View synthesis, media generation, robots, and computational photography.
Mip NeRF 360和即时NGP(iNGP)都基于NeRF的形式:通过投影3D射线并沿着光距离t的位置渲染像素。这些特征被输入到神经网络,渲染后显示颜色。重复投影与训练图像中像素对应的光,并最小化(通过梯度下降)每个像素的渲染颜色与观察到的颜色之间的误差,以完成训练。
Mip-NeRF 360 and instant-NGP(iNGP) are both based on the form of NeRF: pixels are rendered by projecting 3D rays and along the position of light distance t. These features are input to the neural network, and color is displayed after rendering. Repeatedly projecting the light corresponding to the pixels in the training image,And minimize (by gradient descent) the error between the rendering color of each pixel and the observed color to complete the training.
Mip-NeRF 360和即时NGP在沿射线的坐标参数化方面有显著差异。在Mip-NeRF360中,射线被细分为一组区间[t_I,t_I+1],每个区间代表一个圆锥体,其形状类似于多个高斯值,该高斯值的预期位置编码用于大MLP[3]的输入。相比之下,即时NGP将位置的本征值插值到不同大小的三维网格层次中,然后使用一个小的MLP来生成特征向量。作者提出的模型结合了mip-NeRF360的整体框架和即时NGP的特征方法。然而,盲目地直接结合这两种方法会引入两种混叠形式:
Mip-NeRF 360 and instant-NGP have significant differences in coordinate parameterization along rays. In mip-NeRF 360, a ray is subdivided into a set of intervals [t_ I, t_ I +1], each representing a cone, whose shape is similar to multiple Gaussian values,The expected position encoding of this Gaussian value is used for the input of a large MLP [3]. In contrast, instant-NGP interpolates the eigenvalues of positions into a three-dimensional mesh hierarchy of different sizes, and then uses a small MLP to generate eigenvectors. The model proposed by the authors combines the overall framework of the mip-NeRF360 and the characteristic method of the instant-NGP,However, blindly and directly combining these two methods will introduce two aliasing forms:
1.即时NGP特征网格方法与mip-nerf360比例传感集成位置编码技术不兼容。因此,即时NGP生成的特征相对于空间坐标是别名,从而生成别名渲染。在下面的介绍中,研究人员通过引入类似的多采样解决方案来计算预过滤的瞬时NGP特性,从而解决了这个问题。 2.即时NGP的使用显著加速了训练,但它暴露了在线蒸馏方法mip-nerf360的一个问题,这导致了高度可见的“z混叠”(沿光线的混叠),其中场景内容随着相机的移动而不稳定地消失。在下面的介绍中,研究人员使用了一种新的损失函数来解决这个问题,该函数在在线蒸馏过程中沿着每条射线进行预过滤。
1. The instant-NGP feature mesh method is incompatible with the mip-nerf360 scale sensing integrated position coding technology. Therefore, the features generated by instant-NGP are aliases relative to spatial coordinates, thus generating an alias rendering. In the following introduction,Researchers solved this problem by introducing a similar multi-sampling solution for calculating the instant-NGP characteristics of prefiltering.
2. The use of instant-NGP significantly accelerates the training, but it exposes a problem mip-nerf360 the online distillation method, which leads to highly visible “z-aliasing” (aliasing along the ray), in which the scene content disappears unsteadily with the movement of the camera.In the following introduction, researchers use a new loss function to solve this problem, which prefilters along each ray during online distillation.
方法概述 1.空间消除混叠: Mip-NeRF中使用的特征类似于由子元素的内部坐标位置编码的积分,并且在NeRF中是沿着圆锥的圆锥。这导致当每个正弦曲线的周期大于高斯曲线的标准偏差时,Fu Liye特征的幅度非常小,这些特征仅代表子体积在大于子体素大小的波长下的空间位置。由于该特征同时编码位置和尺度,使用它的MLP可以学习呈现抗锯齿图像的3D场景的多尺度表示。像iNGP这样的基于网格的表示不查询子体素,而是在单个点使用三线性插值来构造MLP的特征,这将导致训练的模型无法推断不同的尺度或别名。
Method overview
1.Spatial Anti-Aliasing:
The feature used in the Mip-NeRF is similar to the integral encoded by the position of the internal coordinates of the child element, and in NeRF is a cone along a cone. This results in that when the period of each sine curve is greater than the standard deviation of Gaussian curve, the amplitude of the Fu Liye features is very small-these features only represent the spatial position of the sub-volume at the wavelength greater than the size of the sub-voxel.Because this feature encodes position and scale at the same time, the MLP using it can learn the multi-scale representation of 3D scenes presenting anti-aliasing images. Grid-based representations like iNGP do not query subvoxels, but use trilinear interpolation at a single point to construct features for MLP,This will cause the trained model to be unable to infer different scales or aliases.
为了解决这个问题,研究人员使用多重采样和特征加权,将每个圆锥体变为一组各向同性高斯:首先将各向同性语素转换为一组点来近似其形状,然后将每个点视为各向同性高斯尺度。这种各向同性假设可以利用网格中的值为零均值的事实来近似特征网格在子体素上的实积分。通过对这些加权后的特征进行平均,具有尺度感知的预过滤特征是从iNGP网格中获得的。有关可视化的更多信息,请参阅下图。
In order to solve this problem, the researchers changed each cone into a set of isotropic Gauss, using multi-sampling and feature weighting: the isotropic morphemes are first converted into a set of points to approximate its shape, and then each point is considered as an isotropic Gaussian scale. This isotropic assumption can take advantage of the fact that the value in the mesh is zero mean to approximate the real integral of the feature mesh on the sub-voxel.By averaging these downweighted features, pre-filtering features with scale perception are obtained from the iNGP grid. For more information about visualization, see the following figure.
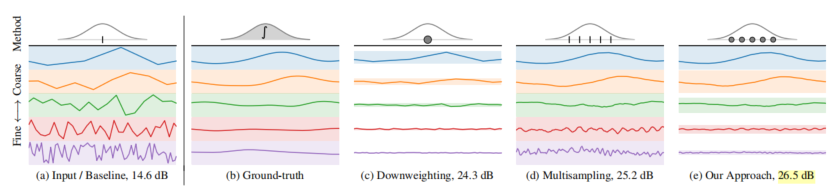
抗锯齿问题在一些图形文献中已经得到了深入的讨论。Mip-map(Mip-nerf同名)预先计算出一种可以快速消除锯齿的结构,但目前尚不清楚如何将这种方法应用于iNGP底部的哈希数据结构。超采样技术采用了直接增加样本数量的方法来抵御混叠,产生了大量不必要的样本。这种方法类似于mip-map,但成本更高。多采样技术构建一组样本,然后将这些样本的信息聚合到聚合表示中,这提供了一个复杂的渲染过程——一种类似于作者方法的策略。另一种相关方法是椭圆加权平均,其类似于沿椭圆长轴排列的各向同性样品的椭圆核。
The problem of anti-aliasing has been deeply discussed in some graphic literature. Mip-map(Mip-nerf name with the same name) pre-calculates a structure that can quickly reverse aliasing, but it is not clear how to apply this method to the hash data structure at the bottom of iNGP. Hypersampling technology adopts a method of directly increasing the number of samples to resist aliasing,A large number of unnecessary samples are generated. This method is similar to mip-map, but costs more. The Multi-sampling technology constructs a group of samples and then aggregates the information of these samples into the aggregate representation, which provides a complex rendering process-a strategy similar to the author’s method.Another correlation method is the elliptic weighted average, which is similar to the elliptic nucleus of an isotropic sample arranged along the long axis of the ellipse.
给定沿射线的间隔[t_I,t_(I+1)),研究人员希望构建一组近似圆锥形的多样本形状。就像在有限样本预算的图形应用程序的多样本程序中一样,他们为自己的用例手动设计了一个多样本模式,沿着螺旋分配n个点,它使m个点围绕射线轴循环,并沿着t形成线性间隔:
Given the interval along the ray [t_ I, t_ (I +1)), the researchers want to construct a set of multi-sample shapes approximately conical. Just as in the multi-sampling program of graphic application with limited sample budget, they manually designed a multi-sampling mode for their use cases, allocating n points along a spiral,It makes m points circulate around the axis of the Ray and form a linear interval along t:
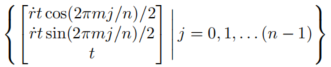
这些三维坐标被旋转成世界坐标,乘以标准正交基。这个标准正交基的第三个向量是光线的方向,它的前两个向量是垂直于视图方向的任何帧,然后按射线的原点移动。当n≥3,n和m是公共素数时,确保每组多个样本的平均值和协方差与每个样本的平均数和协方差精确匹配,类似于mip-NeRF中的高斯采样。
These three-dimensional coordinates are rotated into world coordinates, multiplied by a standard orthogonal basis. The third vector of this standard orthogonal basis is the direction of the ray, and its first two vectors are any frame perpendicular to the direction of the view, and then move by the origin of the ray. When n≥3 and n and m are common prime numbers, ensure that the mean and covariance of each group of multiple samples exactly match the mean and covariance of each sample,Similar to Gaussian Sampling in mip-NeRF.
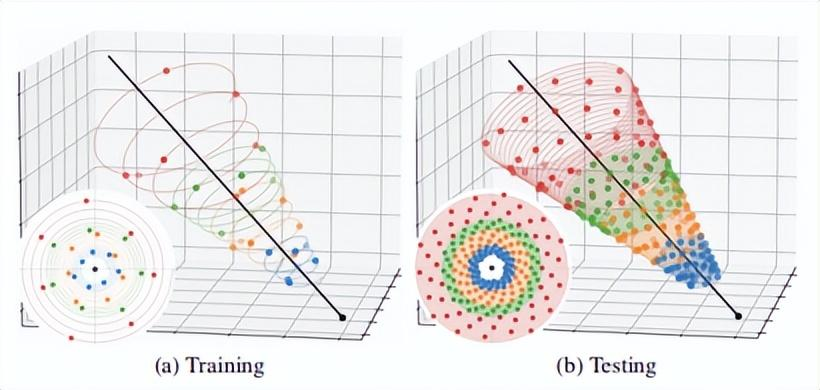
研究人员使用这n个多重样本作为各向同性高斯分布的平均值,每个样本的标准偏差为__J。他们将speech_J设置为rt,并传递一个超级参数(实验中为0.35)。因为iNGP网格要求输入坐标位于有界区域,研究人员应用了mip-NeRF 360的收缩函数。由于这些高斯分布是各向同性的,我们可以使用mip-NeRF360使用的卡尔曼滤波器方法的简化和优化版本来执行这种收缩。有关更多信息,请稍后添加。 为了对每个单个多样本进行逆别名插值,研究人员以一种新的方式重新加权每个尺度上的特征,这与每个网格单元中每个样本的各向同性高斯拟合度成反比:如果高斯值远大于插值单元,插值特征可能是不可靠的,那么应该减少权重。Mip-NeRF的IPE特征也有类似的解释。 在iNGP中,每个坐标x的插值是通过缩放网格的线性大小n来实现的,并对V_l进行三线性插值以获得c长度向量。相反,研究人员对一组平均值和标准偏差为_J的多采样各向同性高斯分布进行插值。通过对高斯CDF的推断,我们可以计算出V中[1/2n,1/2n]^3内每个高斯PDF的分数,该分数被插值为尺度相关的递减权重因子ω_J,l.研究人员应用权重衰减来鼓励V值符合正态分布和零均值。现单个零制服等L,可能的项目总数。
Researchers used these n multiple samples as the mean of isotropic Gaussian distribution, and the standard deviation of each sample was__J. They set speech_j to rt and passed a super parameter (0.35 in the experiment). Because the iNGP grid requires the input coordinates to be located in a bounded area,Researchers applied the contraction function of mip-NeRF 360. Because these Gaussian distributions are isotropic, we can use the simplified and optimized version of the Kalman filter method used by mip-NeRF 360 to perform this contraction. For more information, please add it later.
In order to carry out inverse alias interpolation for each single multi-sample, researchers re-weighted the features on each scale in a new way, which is inversely proportional to the isotropic Gaussian fitting degree of each sample in each grid cell: If the Gaussian value is far greater than the interpolation cell, the interpolation features may be unreliable, then the weighting should be reduced.The IPE features of Mip-NeRF are similarly explained.
In iNGP, the interpolation of each coordinate x is achieved by scaling with the linear size n of the mesh, and conducting trilinear interpolation on V_l to obtain a c-length vector. On the contrary, researchers interpolate a set of multi-sampling isotropic Gaussian distributions with mean and standard deviation of_J.Through the inference of Gaussian CDFs, we can calculate the fraction of each Gaussian PDF within [1/2n,1/2n]^ 3 in V, which is interpolated into a scale-related decreasing weight factor ω_j,l. Researchers apply weight attenuation to encourage the value in V to conform to normal distribution and zero mean.现 Individual zero-uniform, etc. L., Possible total number of items.

尽管前面提到的精细多采样和加权方法是减少空间混叠的有效方法,但我们必须考虑到沿着光z混叠有一个额外的混叠源。这是因为MLP学习在使用mip-NeRF360的情况下生成上限场景几何体:在训练和渲染期间,通过沿射线重复评估该MLP生成的直方图的下一轮采样,只有最后一组样本由NeRF MLP网络呈现。Mip-NeRF 360表明,与之前学习一个或多个NeRF的策略相比,该方法显著提高了速度和渲染质量,并且这些策略都使用图像重建损失来监督。研究人员发现,mip-NeRF 360中的MLP方案倾向于学习从输入坐标到输出体积密度的非平滑映射。这将导致光线跳跃的场景内容的阴影,如上图所示。尽管这种错觉在mip NeRF 360中非常小,但如果作者在他们提出的网络中使用iNGP后端而不是MLP(这可以提高新模型的快速优化能力),它就会变得常见和视觉突出,尤其是当相机沿其Z轴切换时。
Although the fine multi-sampling and weighting methods mentioned earlier are effective methods to reduce spatial aliasing, we must consider that there is an additional aliasing source along the light-z-aliasing. This is because MLP learning generates upper limit scene geometry under the use of mip-NeRF360: during training and rendering,The next round of sampling of the histogram generated by repeatedly evaluating this MLP along the ray, only the last group of samples is presented by the NeRF MLP network. Mip-NeRF 360 indicates that compared with the previous strategy of learning one mi-nerf or more nerf,This method significantly improves the speed and rendering quality, and these strategies all use image reconstruction loss to supervise. Researchers found that the MLP scheme in mip-NeRF 360 tends to learn non-smooth mapping from input coordinates to output volume density. This will cause a shadow of the scene content of a ray jump,As shown in the image above. Although this illusion is very small in mip-NeRF 360, if the authors use iNGP backend instead of MLP in their proposed network (which can increase the rapid optimization capability of the new model), it becomes common and visually prominent, especially when the camera switches along its Z axis.
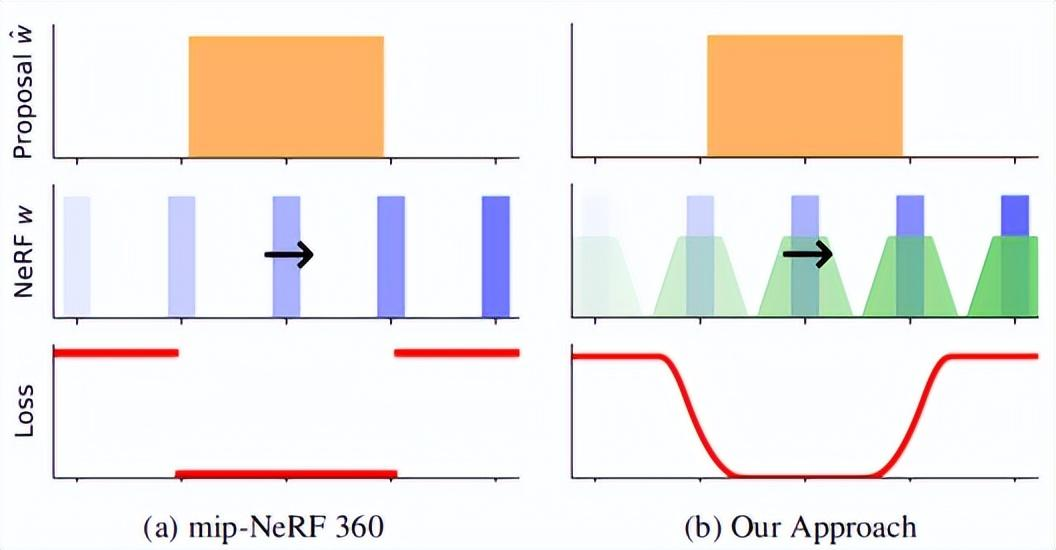
在下图中,研究人员可视化了训练实例的提案监督,其中窄的NeRF直方图(蓝色)相对于粗略的提案直方图(橙色)沿着射线平移。(a)mip-NeRF360使用的损失是分段常数,但(B)新模型的损失是平滑的,因为研究人员将NeRF直方图模糊为分段线性样条(绿色)。新模型中的预滤波损失可以学习抗锯齿的提议分布。 消除混叠交错损失:
In the following figure, researchers visualize the proposal supervision of a training instance, in which a narrow NeRF histogram (blue) is translated along a ray relative to a rough proposal histogram (orange). (a) the loss used by the mip-NeRF360 is a piecewise constant,But (B) the loss of the new model is smooth because researchers blur the NeRF histogram into piecewise linear splines (green). The pre-filtering loss in the new model can learn the proposal distribution of anti-aliasing.
Anti-Aliased Interlevel Loss:
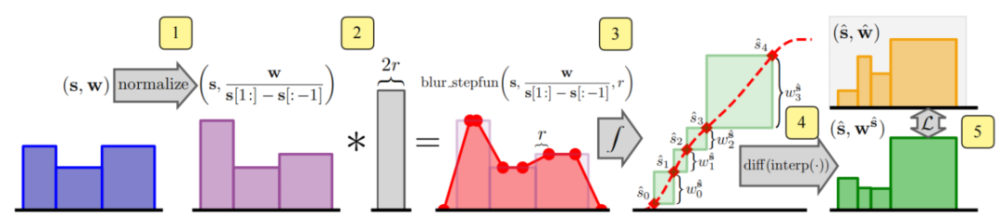
研究人员继承的mip NeRF 360中的提案监督方法需要一个损失函数,该函数以NeRF生成的阶跃函数(s,w)和提案模型生成的类似阶跃函数为输入。这两个阶跃函数是直方图,中间是权重的权重,其中,w_i显示可能的风景内容函数区间i。(S_i)研究人员稍后将对此进行讨论。请注意,S和语音是不同的,每个直方图的端点是不同的。
The proposal supervision method in mip-NeRF 360 inherited by researchers requires a loss function, which takes the step function generated by NeRF(s,w) and the similar step function generated by proposal model (^ s,^ w) as input. These two step functions are histograms,In the middle, the weight of the weight of the weight, among them, w_i display possible scenery content function interval I. (S_i)Researchers will discuss it later. Note that s and speechs are different-the endpoints of each histogram are different.
网络绑定场景的几何预测的训练建议NeRF不会引入混叠。研究人员需要一个可以测量距离(s,w)和(BeautsŞw)的损失函数来平滑这对射线,尽管这两个步骤的端点函数不同。为此,研究人员将使用他们预先构建的算法来模糊NeRF直方图(s,w),然后,将模糊分布重新采样到提议直方图对数的区间集,以生成一组新的直方图权重。
Training proposal NeRF of geometric prediction of network-bound scenarios does not introduce aliasing. Researchers need a loss function that can measure distances (s,w) and (Beauts ˆ w) to smooth the pair of rays, although the endpoint functions of these two steps are different. To do this, researchers will use their pre-built algorithm to blur the NeRF histogram (s,w),Then, the fuzzy distribution is re-sampled to the interval set of proposal histogram logariths to generate a new set of histogram weights.
这个过程如上图所示。将模糊NeRF权重重新采样到提案的直方图空间后,模型的损失函数是总和w的元素级函数,如下所示:
This process is shown in the preceding figure. After the fuzzy NeRF weight is resampled to the histogram space of the proposal, the loss function of the model is the element-level function of the sum w, as follows:

Normalizing Metric Distance:
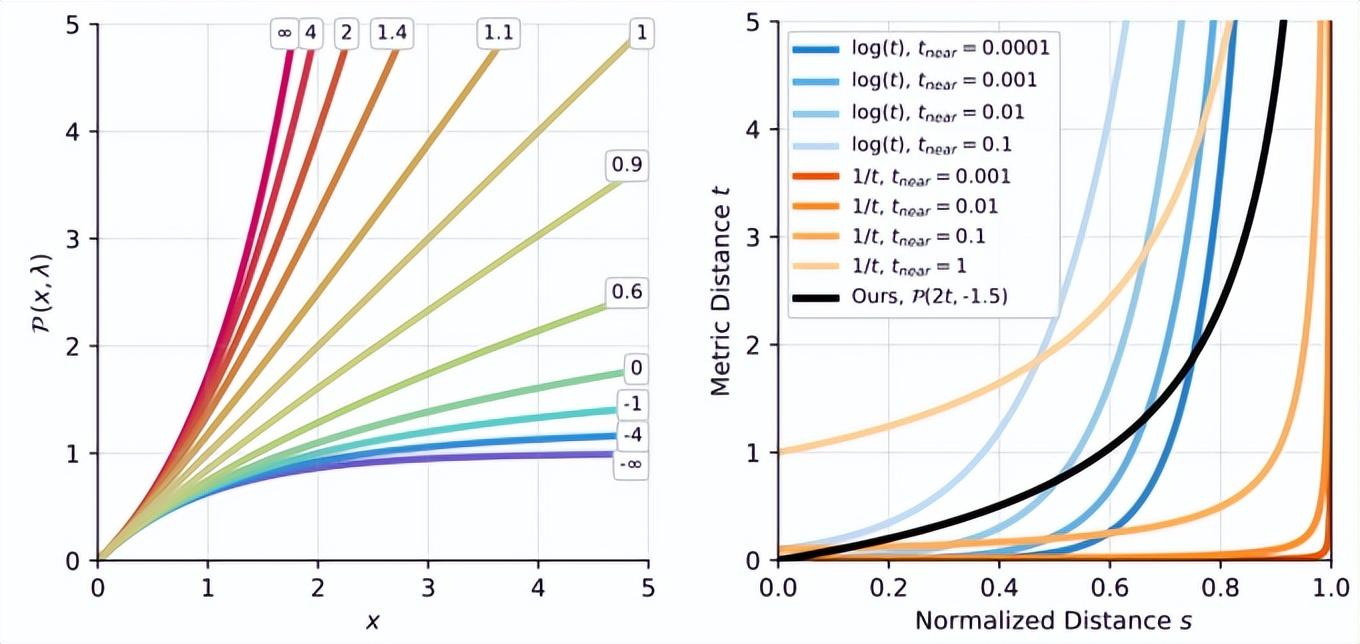
许多NeRF方法需要一个函数来将测量距离t∈[0,∞)转换为标准化距离s∈[0,1]。左:功率变换P(x,λ)允许通过修改λ(如线性、对数和倒数)在公共曲线之间进行插值,同时在原点附近保持线性形状。右:构建从线性过渡到反向/反向查询的曲线,并支持靠近相机的场景内容。
Many NeRF methods require a function to convert the measurement distance t∈[0,∞) to the standardized distance s∈[0,1]. Left: power transformation P(x,λ) allows interpolation between common curves by modifying λ, such as linearity, logarithm and inverse, while maintaining a linear shape near the origin.Right: construct a curve from linear transition to inverse/reverse query, and support the scene content close to the camera.
实验结果: 研究人员的模型在JAX中实现,并在基线360的mip-NeRF的基础上,重新设计和实现了iNGP的体素网格和哈希表结构,取代了360使用的mip-NelF大型MLP网络,整体模型架构与mip NeRF 360相同。 360数据集多尺度版本的性能,多尺度图像的训练和评估。红色、橙色和黄色高亮显示代表每个指标的第一、第二和第三最佳性能技术。所提出的模型显著优于两个基线,尤其是基于iNGP的基线,新模型的误差减少了54%-76%。A-M线是模型的烧蚀实验。有关详细信息,请参阅论文末尾的扩展文本。
Experimental results
The researchers’ model was implemented in JAX, and based on the mip-NeRF of baseline 360, the voxel grid and hash table structure of iNGP were redesigned and implemented, replacing mip-NeRF large MLP network used by 360. In addition to the anti-aliasing adjustment introduced in it,The overall model architecture is the same as that of mip-NeRF 360.
Performance on the multi-scale version of 360 Datase, training and evaluation of multi-scale images. Red, Orange, and yellow highlights represent the first, second, and third best performance techniques for each indicator. The proposed model is significantly superior to two baselines-especially iNGP-based baselines,Especially on rough scale, the error of the new model is reduced by 54%-76%. Line A- M is the ablation experiment of the model. For details, please refer to the extended text at the end of the paper.

尽管360dataset包含许多具有挑战性的场景内容,但它无法将渲染质量作为尺度的函数来测量,因为该数据集是通过在大致恒定的距离处围绕中心对象拍摄相机来获得的,并且学习模型不需要在不同的图像分辨率或距离下处理和训练中心对象。因此,研究人员使用了一个更具挑战性的评估过程,类似于使用mip-NeRF多尺度混合器数据集:研究人员将每张图像更改为一组四张图像,分别以[1,2,4,8]的比例进行下采样。额外的训练/测试视图相机已从场景中心放大。在训练过程中,研究人员将数据项乘以每条射线的比例因子,并在测试过程中分别评估每个比例。这大大增加了模型跨尺度泛化的重建难度,并导致混叠伪影的明显出现,尤其是在粗尺度上。 在表1中,研究人员基于iNGP、mipNeRF 360、mip NeRF 360+iNGP基线和许多消融方法对新提出的模型进行了评估。尽管mip NeRF360表现合理(因为它可以训练多个尺度),但新模型在最精细的尺度上降低了8.5%,在最粗糙的尺度上减少了17%,mip-NeRF 360+iNGP基线性能较差,因为它没有抗混叠或推理尺度机制:新模型的均方根误差在最精细的尺度下降低了18%,在最粗糙的尺度下下降了54%,在最粗糙的尺度下,DSSIM和LPIPS降低了76%。这一改善可以在下图中看到。正如他们在第二张表中预期的那样,研究人员的mip-NeRF 360+iNGP基线通常优于iNGP(除了最厚的尺度)。
Although 360dataset contains many challenging scene contents, it cannot measure the rendering quality as a function of scale, because this dataset is obtained by shooting the camera around a central object at a roughly constant distance, and the learning model does not need to process and train the central object at different image resolutions or distances.Therefore, researchers used a more challenging evaluation process, similar to using mip-NeRF multi-scale blender data sets: Researchers changed each image into a set of four images which were downsampled with [1,2,4,8] scales respectively. Additional training/test View cameras have been magnified from the center of the scene.During the training, the researchers multiplied the data item by the scale factor of each ray, and they evaluated each scale separately during the test. This greatly increases the reconstruction difficulty of cross-scale generalization of the model, and leads to the obvious occurrence of aliasing artifacts, especially on the coarse scale.
In Table 1, researchers evaluated the newly proposed model based on iNGP, mipNeRF 360, mip-NeRF 360 + iNGP baseline and many ablation methods. Although mip-NeRF 360 behaves reasonably (because it can train multiple scales),The new model reduced by 8.5% on the most delicate scale, 17% on the roughest scale, and 22 times faster at the same time. mip-NeRF 360 + iNGP baseline has poor performance because it has no anti-aliasing or inference scale mechanism: the root mean square error of the new model is 18% lower at the most refined scale,It is 54% lower in the roughest scale, and 76% lower in DSSIM and LPIPS in the roughest scale. This improvement can be seen in the following figure. The mip-NeRF 360 + iNGP baseline of researchers is generally better than iNGP (except for the thickest scale),As they expected in the second table.
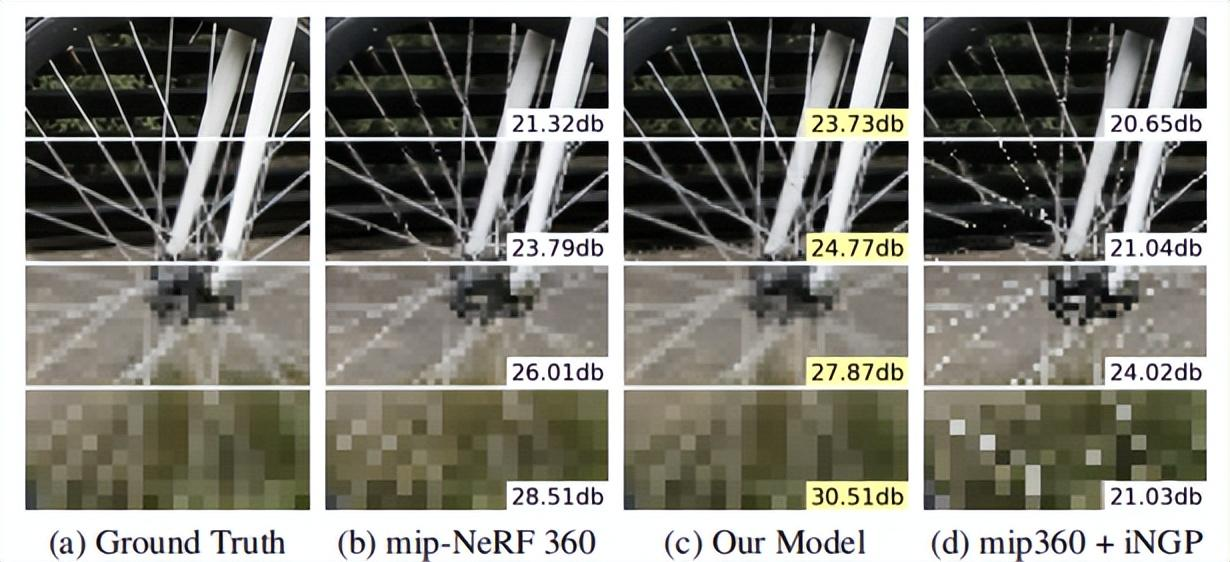
总结: 研究人员提出了Zip-NeRF模型,该模型融合了尺度感知抗混叠NeRF和基于快速网格的NeRF训练两种方法的优势。通过使用多采样和预滤波的方法,该模型可以实现比以前技术低8%-76%的错误率。同时,它比mip-NeRF360(目前解决相关问题的最先进技术)快22倍。研究人员希望本文提出的关于混叠的工具和分析(网络的空间混叠是从空间坐标的颜色和密度映射而来的,z混叠的损失函数是沿着每条射线在线提取的)可以进一步提高了nerf逆绘制技术的质量、速度和成品效率。
Summary
Researchers have proposed Zip-NeRF model, which integrates the advantages of two methods of scale sensing anti-aliasing NeRF and NeRF training based on fast grid. By using the method of multi-sampling and pre-filtering, the model can achieve an error rate of 8%-76% lower than that of the previous technology,At the same time, it is 22 times faster than mip-NeRF360 (the most advanced technology for current related problems). Researchers hope that the tools and analysis proposed here about aliasing (the spatial aliasing of the net is mapped from the color and density of spatial coordinates, and the loss function of z-aliasing is distilled along each ray online) can further improve the quality of nerf inverse rendering technology,Speed and finished product efficiency.
全部评论(0)